Understanding Representation in Artificial Intelligence
JM
“The key to artificial intelligence has always been the representation.” - Jeff Hawkins
Understanding Representation in Artificial Intelligence
As artificial intelligence (AI) continues to infiltrate various aspects of our daily lives, understanding how representation works within these systems becomes increasingly important. Representation in AI refers to how information, concepts, and knowledge are structured and processed by machines. This foundational aspect plays a crucial role in determining how effectively AI systems can interpret, learn from, and interact with the world.
At its core, representation involves encoding real-world information into a format that an AI system can use to make decisions. This process is critical because an AI's capabilities are largely determined by how well it can understand and manipulate the data it receives. From basic image recognition to complex natural language processing, every AI application relies on effective representation.

The Importance of Data Representation
Data representation is fundamental in AI as it directly impacts the accuracy and efficiency of an AI system. It involves transforming raw data into a format that can be easily processed by algorithms. This transformation is necessary because raw data is often too complex or unstructured for an AI to handle without modification.
For instance, in image recognition, data representation might involve converting a visual scene into numerical values that describe various features such as shapes, colors, and textures. This process allows the AI to create a model of the image that can be analyzed and compared with other data. The quality of this representation affects how well the AI can recognize patterns and make predictions.
Types of Representation Methods
There are several methods used for representation in AI, each suited to different types of data and applications. Some common methods include:
- Semantic Networks: These are graphical representations of knowledge that showcase relationships between concepts, acting like a map of interconnected ideas.
- Neural Networks: Inspired by the human brain, these models use layers of interconnected nodes to represent complex patterns and relationships in data.
- Decision Trees: A hierarchical model that represents decisions and their possible consequences, making it useful for classification tasks.
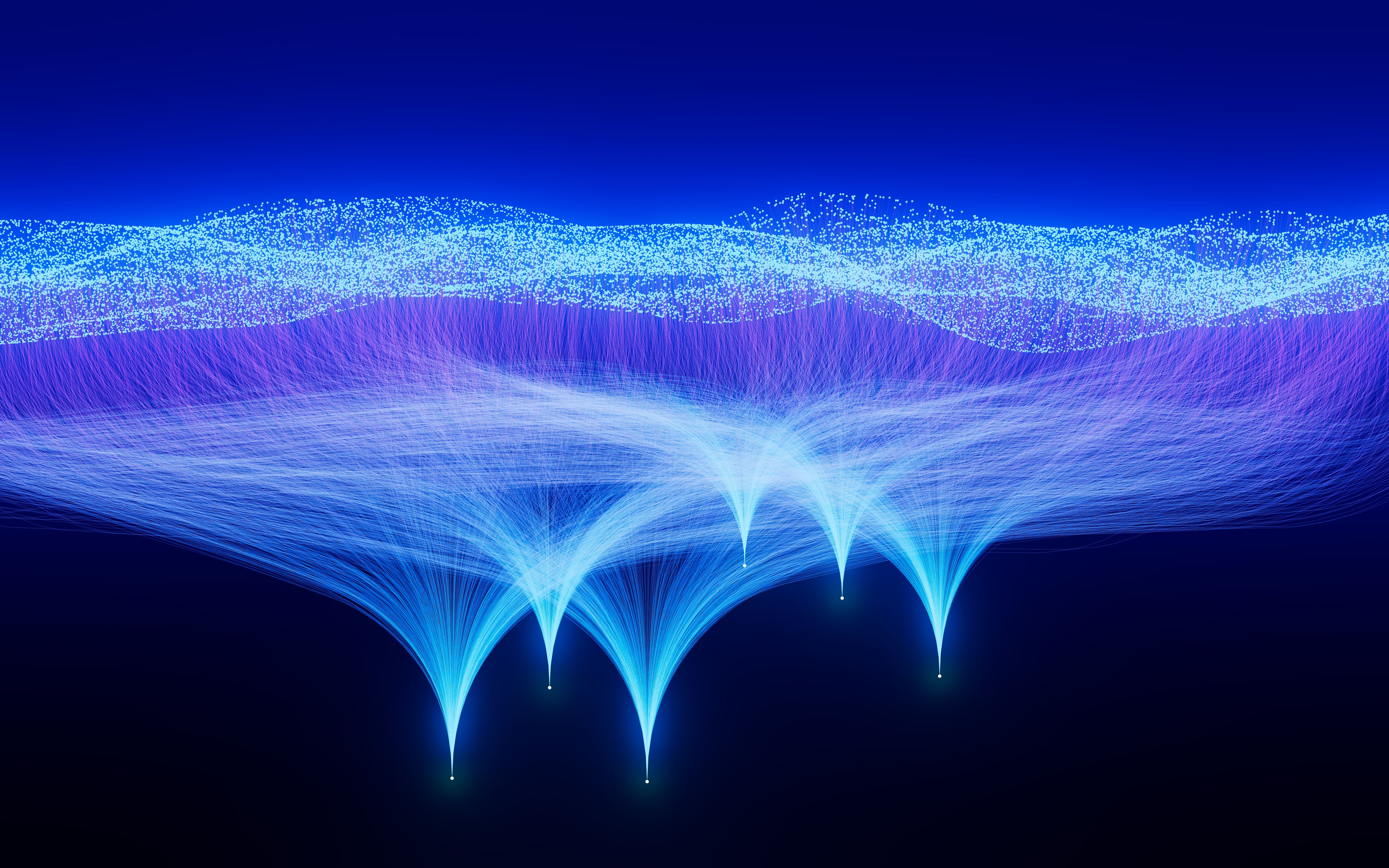
Challenges in Representation
One of the biggest challenges in AI representation is ensuring that the chosen method accurately reflects the complexity of real-world information. Poor representation can lead to biases, inaccuracies, or inefficiencies in how an AI system functions. This is particularly concerning in areas like healthcare or criminal justice, where decisions made by AI can have significant consequences.
Another challenge is scalability. As the volume of data increases, maintaining an efficient and accurate representation becomes more difficult. AI systems must adapt to handle more information without compromising performance, which requires ongoing research and innovation.
The Role of Representation in Machine Learning
In machine learning, representation is crucial for training algorithms to recognize patterns and make decisions. The quality of a machine learning model heavily relies on how well the input data is represented. Effective representation can enhance a model's ability to generalize from training data to new, unseen scenarios.
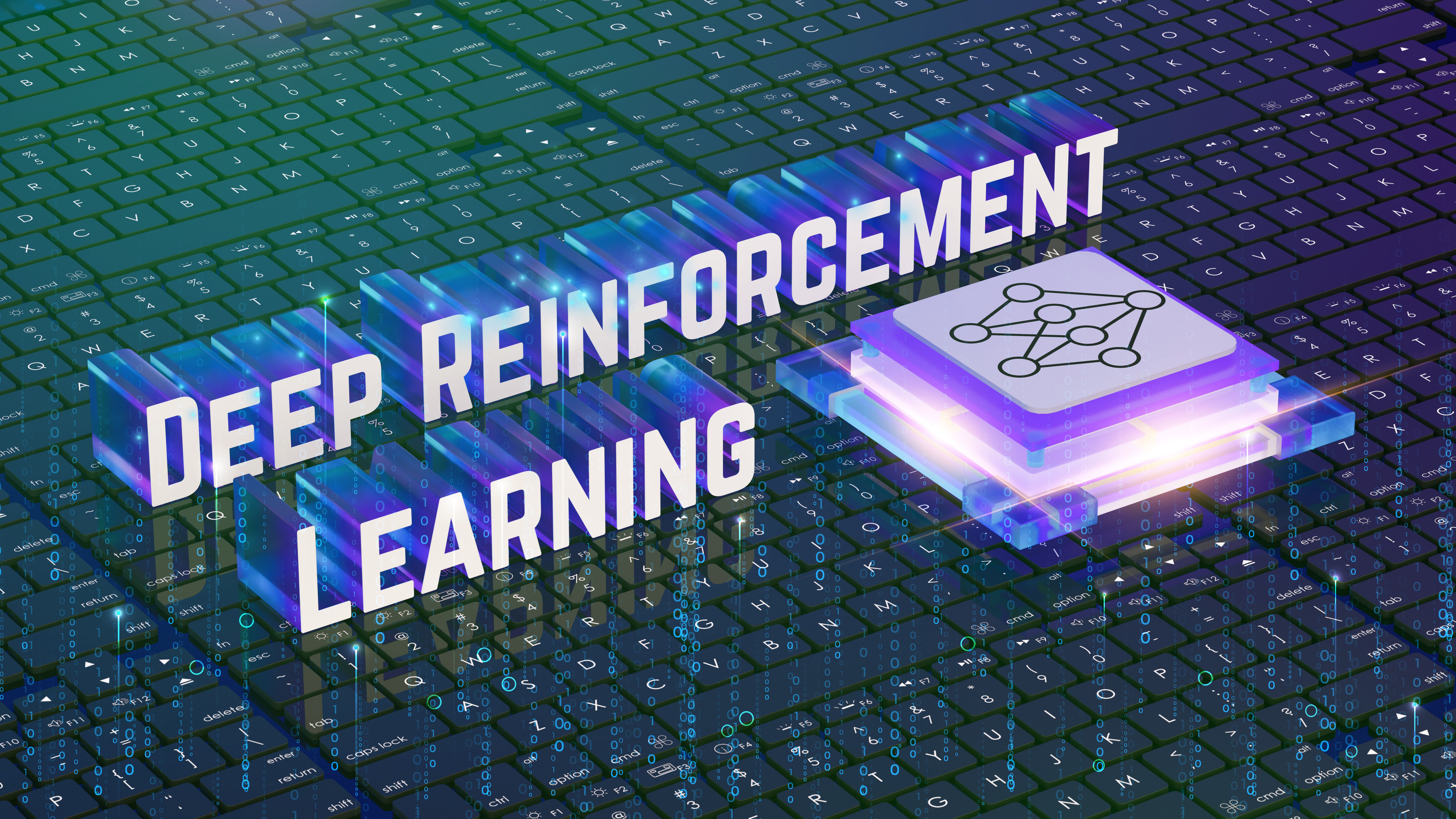
Moreover, advancements in representation learning are enabling AI systems to automatically discover the best way to represent data without extensive manual intervention. This area of research holds promise for improving the flexibility and applicability of AI across diverse domains.
Conclusion
Understanding representation in artificial intelligence is vital for developing systems that are both effective and ethical. As AI continues to evolve, ongoing efforts to refine representation methods will play a key role in unlocking new capabilities and ensuring that technology serves the needs of society responsibly. By focusing on accurate, efficient, and fair representations, we can harness the full potential of AI while mitigating risks associated with its deployment.